Dear All,
I would like to thank you Elsmar community and wish to you all happy an safe new year 2021!
We have done visual inspection for one of our client before goods are shipped. Based on the result "Pass", our client allowed the shipment.
5 lots are concerned. Each inspection is done on various date. We inspected 200 samples (Level II) from 5 differents lots.
Our client came back to us telling they have discovered 1.48% defective rate on the shipment inspected by our team and statistically speaking there was only 5.07% of probability to accept the batch with this defect ratio.
We don inspection by attributes and have 3 types of defect classification :
Sampling size: Level II Normal
Critical: 0.065%
Major: 2.5%
Minor: 4.0%
If on a single piece we have multiple kind of defect, we will consider the most serious one as rejection reason: Critical > Major > Minor
Our client found as above around 1.48% of critical defective rate on the totality of pieces received: open seam
Our team didn't found this type of defect on inspected pieces. We assume they did the inspection properly as they have found other type of defects.
Our client is aware of the %P(a) of 5.07% of accepting a bad lot based on the OC Curve. But only for 1 inspection and says this is not normal to not having found at least 1 defect on the 5 different inspections.
I argued the same: probability but still don't agree.
Some will tell: if your client doesn't agree, he just switch to 100% inspection
Some will tell: this is the nature for the acceptance sampling and the risk "0" doesn't exist.
I tried to explain that for a lot of 4080 pieces we need to realise appr. 21 inspections of 200 pieces to inspect 100% of the lot. As we talk about probabilities, we can never know on which of 21 inspections we will detect these 2.06% defective units.
I tried to illustrate roughly the defected unit distribution and the risk involved to our client. But, still not conviced.
My questions are:
* How I can calculate the probability of falling on good / bad samplings among 21 inspections?
If there is only 1.56% of a probability to not detect at least 1 defect in the sample size of 200 pieces with c=0 and %p = 2.06% there should be also a way of calculating the probability of not fall on the sample size with at least 1 defect.
* Why the Lot size is not taken into account?
I would really appreciate your help,
I would like to thank you Elsmar community and wish to you all happy an safe new year 2021!
We have done visual inspection for one of our client before goods are shipped. Based on the result "Pass", our client allowed the shipment.
5 lots are concerned. Each inspection is done on various date. We inspected 200 samples (Level II) from 5 differents lots.
Our client came back to us telling they have discovered 1.48% defective rate on the shipment inspected by our team and statistically speaking there was only 5.07% of probability to accept the batch with this defect ratio.
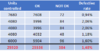
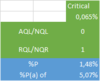
We don inspection by attributes and have 3 types of defect classification :
Sampling size: Level II Normal
Critical: 0.065%
Major: 2.5%
Minor: 4.0%
If on a single piece we have multiple kind of defect, we will consider the most serious one as rejection reason: Critical > Major > Minor
Our client found as above around 1.48% of critical defective rate on the totality of pieces received: open seam
Our team didn't found this type of defect on inspected pieces. We assume they did the inspection properly as they have found other type of defects.
Our client is aware of the %P(a) of 5.07% of accepting a bad lot based on the OC Curve. But only for 1 inspection and says this is not normal to not having found at least 1 defect on the 5 different inspections.
I argued the same: probability but still don't agree.
Some will tell: if your client doesn't agree, he just switch to 100% inspection
Some will tell: this is the nature for the acceptance sampling and the risk "0" doesn't exist.
I tried to explain that for a lot of 4080 pieces we need to realise appr. 21 inspections of 200 pieces to inspect 100% of the lot. As we talk about probabilities, we can never know on which of 21 inspections we will detect these 2.06% defective units.

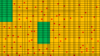
I tried to illustrate roughly the defected unit distribution and the risk involved to our client. But, still not conviced.
My questions are:
* How I can calculate the probability of falling on good / bad samplings among 21 inspections?
If there is only 1.56% of a probability to not detect at least 1 defect in the sample size of 200 pieces with c=0 and %p = 2.06% there should be also a way of calculating the probability of not fall on the sample size with at least 1 defect.
* Why the Lot size is not taken into account?
I would really appreciate your help,